Embrace Equity in AI/ML
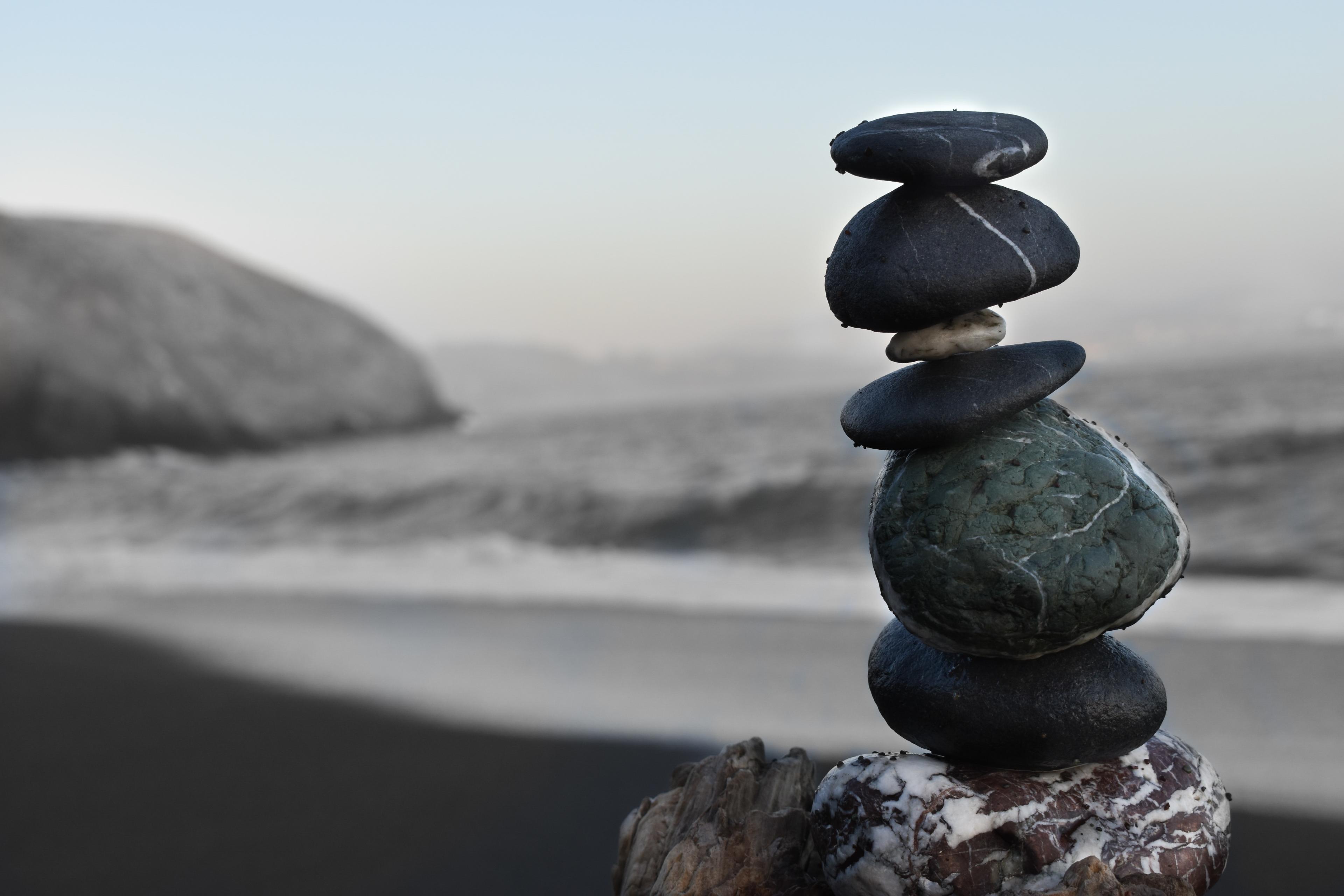
Photo by Colton Sturgeon on Unsplash
What Do We Mean by Equity?
Well, to me equity isn't about all things being the same for everyone, rather than the opportunities are the same for everyone. Representation matters. Outcomes matter. But that doesn't mean that the way to achieve equitability is through equal distribution of the same resources. Different needs should be tended to appropriately to achieve equity.
Why Does Equity Matter in AI/ML?
Those experienced in AI/ML will know (and those starting off in AI/ML should know) that our models can only be as good as the data they are trained on. In line with that, our models can really only make robust inferences about the data they are trained on. In the end, our models don't know or care about the real world context, they just see the data objectively and learn solely based on that. It's for us as the humans writing these models to provide the context and check the decision making/inferences of the models with this in mind.
We have a choice as people choosing to write AI/ML models, to put equity at the heart of the models we create or not. Either way we are shaping the world around us. Let me be more concrete about this...
A Healthcare Conundrum..
Say you are working on AI/ML to interpret medical scans, classifying these based on whether a person has a life threatening condition or not. There can't be anything wrong with that, right? Well, no, not in a cursory sense. These models are aiming to help diagnose people so they can get treatment with, let's say, close to 100% accuracy (the dream, right?) Actually, let's be a bit more realistic and say 98.5% overall accuracy. In reality though, you're finding that the model is performing better for some groups compared to others. Maybe 95% accuracy for women, and 99.5% accuracy for men. So you delve further and realise that most of the training data represented Caucasian men.
Hopefully you're seeing the red flags here...Firstly, we are a population of nearly 50/50 men and women...so the training set should be representative of that, no? Humans are not a homogenous dataset, we have variation. A key one is between sexes, but what else should we be thinking about? Well, we vary not just by sex but also by race, social group, living environment etc. The list goes on and on.
Now that's not to say that all these things are super important to consider for every data set and model. Some may be more relevant than others. For this health example for instance, sex is probably important but also maybe ethnicity and other important groups that might add to physiological variety.
Is it okay for the model to only be trained on Caucasian men? Well, the results indicate not. There is a much higher accuracy for men than women. They both seem really high, but putting that in numbers that's 5 in every 100 (5%) women getting misdiagnosed, compared to only 1 in every 200 (0.5%) men - that means women are 10x more likely to be misdiagnosed. Those could be people who get treatment that don't need it, maybe yielding other complications. Or maybe people who need treatment aren't getting it. Are we okay with that? More specifically, are we okay that this misdiagnosis happens more for a particular group? What is the threshold we will accept for bias models? How do we balance overall model performance compared to performance of individual groups?
Hopefully, you're now thinking about those other demographics, how does the model perform for both men and women with a different ethnicity? Well, for this hypothetical example we don't know. Though, maybe this gets you thinking about what potential biases could be and how you might go about spotting them.
What Choice Do We Have?
There are plenty of examples where biased algorithms can cause harm to society. Above is just one example. When we look at how AI/ML might be used in other sectors, the situation poses some questions that we have to decide the answers to..
We've already said that an AI/ML model can only make inferences based on the input data, and also that the input data should be representative of the population. But what if the real-world equivalent of the model yields biased data that is representative of the context? What happens then?
In recent months and years, there has been more of a light shined on biases in our everyday lives. Whether that's in the legal system, workplace, healthcare and beyond. Do we, the people writing the models of the present and future, want to perpetuate and even exaggerate these biases or do we want to alter the algorithms to eliminate the bias altogether? Is there a should in there, along with those wants?
Now, this is a genuine question, like all the others in this blog post. We have choices to make, and with choice comes differences of opinion. Who's to say I'm right about this? I first started thinking about this years before I started as an ML Engineer, thanks to a great (non technical, accessible) book by Hannah Fry named Hello World. If you're interested in this topic, I'd say that's a great place to start.